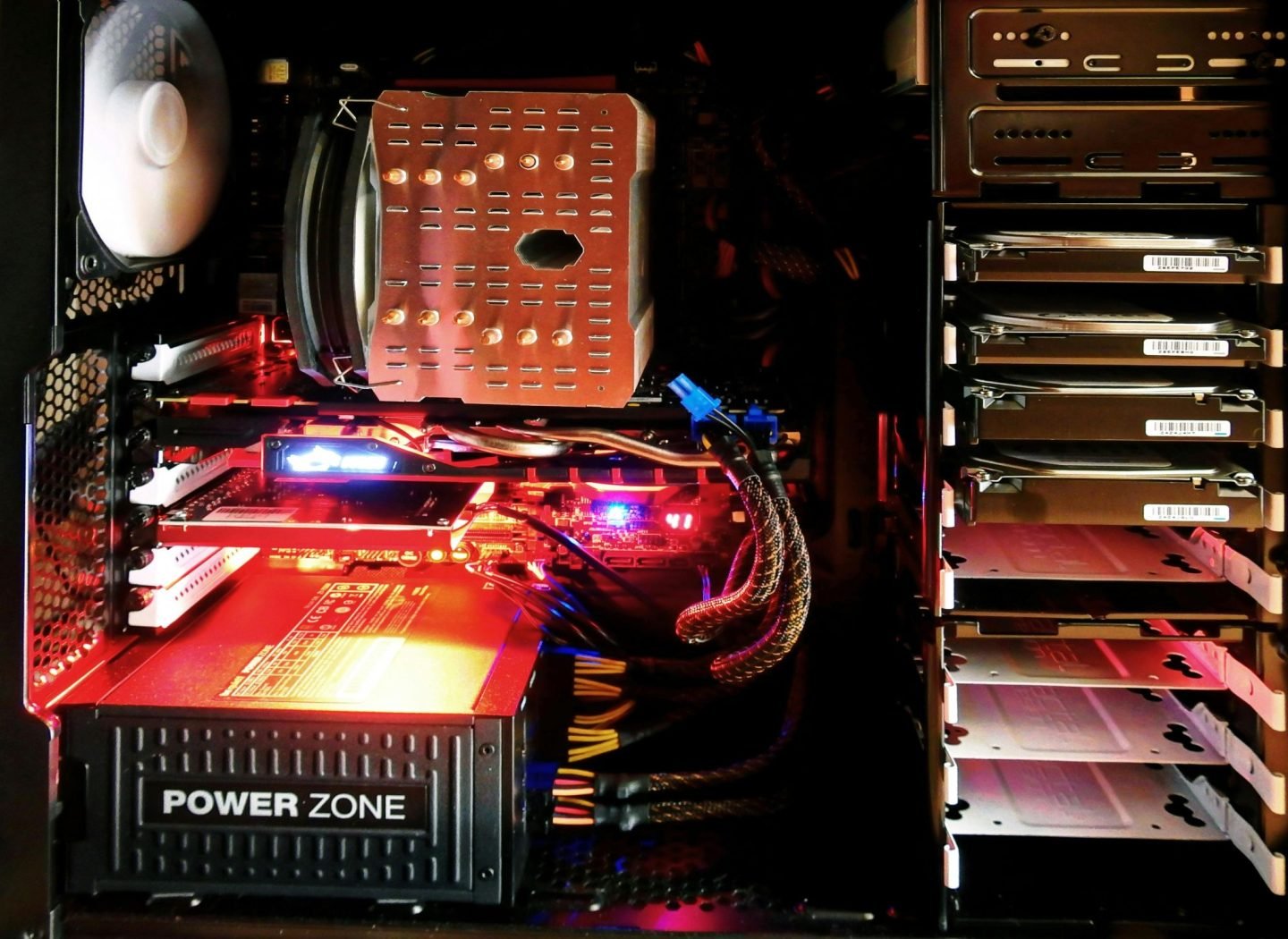
The following piece will get into the nuts and bolts concerning the key elements of constructing a highly effective GPU server case. In today’s climate, the importance of a solid GPU server case has reached an all-time high, especially within the fields of artificial intelligence and cloud and IT infrastructure, as the world continuously embraces the notion of improved performance computing.
For more in-depth information, you should view the GPU Server Chassis – ONECHASSIS.
What is a GPU Server and Why is it Important?
Simply put, a GPU Server is a unit constructed for high-end computing and possesses multiple graphic processing units or GPUs. Typically, when dealing with central processing units, they are able to perform a considerable amount of tasks in a chronological manner with remarkable efficiency. However, a GPU does the opposite and excels in the division of tasks, allowing multiple tasks to be performed simultaneously. This makes it imperative for sectors that require a heavy amount of computing, such as AI and Machine learning.
View GPU Server Chassis – ONECHASSIS for More Details
Role of GPUs within the Realm of High-Performance Computing
GPUs do not perform computations sequentially instead they do it in batches. With the ability to handle numerous parallel processes simultaneously, GPUs are incredibly useful when it comes to tasks which would otherwise be impossible to do for standard systems, such as deep learning and data modelling and analysis. The scalability that GPUs offer help businesses make the most out of real-time simulations and workloads.
Key Features of a GPU Server
Here in this blog, we will look at the Essential Features that a GPU Server should have and most of the machine learning specialists out there would generally prefer a gpu server to have them built in.
Essential Features of A GPU Server:
- Scalable Architecture:
Starting with the first one, a GPU Server should have Scalability since the demand mathematically is bound to increase, Already Moduler’s designs make future hardware enhancements practically effortless. - Rackmount Design:
With the chassis being rackmount compatible, it promotes easiness with data center integration and also ensures Spatial Efficiency, as a result, we closely serve our third essential feature. - Robust Cooling Systems:
GPU’s after a period of usage generate a lot of heat, Take for instance a laptop, The GPU in it when you use games generates significant amount of heat. Multi-stage cooling along with High-performance fans or even Liquid Cooling solutions are some examples. - High Bandwidth Connections:
With PCIe Gen 4 or Gen 5, Communication between components is ensured to be Seamless. - Customizability:
GPU Servers should be flexible in relation to pairing them together with what types of processors, however Intel Xeon or AMD EPYC are great options to pair along with.
Applications of GPU Servers in AI and Machine Learning
GPU servers serve as the core for AI & ML businesses allowing compilation of huge model sets, here is what they do:
Deep Learning Frameworks:
With GPU servers, executing frameworks such as Tensorflow and Pytorch takes less time hence training the model is a quicker process.
Big Data Analysis:
GPU servers are utilized by data scientists to streamline large amounts of data and study it thoroughly.
Cloud Based AI Solutions:
AI services being cloud based require infrastructure GPU Server clusters offer that.
How to Build a Scalable 4U GPU Server
A 4U GPU Server is a server that typically has a height of 4 rack units and is popular, especially in professional settings. Here is how you can build an optimal one:
**Step 1:** Selection of Chassis and Rack form factor.
To begin, choose a chassis that can hold multiple GPUs. A good option for the server is a 4U rack mounting as it is good at holding multiple GPUs because it has the ability to host proper airflow and cable organization.
*Recommendation*: Try and choose a Chassis that supports around 4 to 8 Nvidia A100 GPUs or AMD Instinct accelerators.
**Step 2:** Improved Performance via Integration of Intel Xeon Scalable Processors.
Enhance your GPU Server Performance by pairing it with Intel Xeon Scalable Processors or AMD Threadrippers as they deliver a good balance between the CPU and GPU. This also enables better parallel workload optimization and data exchange.
**Step 3:** Don’t Forget the Power and Cooling Requirements for GPGPU tasks.
High end GPU’s in particular Nvidia RTX A6000 or AMD Radeon Instinct MI210 GPUs have power requirements of over 300 watts each. to be on the safe side, get redundant PSUs (Power Supply Units) and high end cooling technologies.
Power: A multi-12V rail system can ensure stable energy distribution.
Cooling: Hybrid water cooling solutions are effective for sustained gaming or ML workloads.
Why Consider a High-Performance GPU Server for AI Development?
Boosting AI Training Efficiency with GPUs
Graphics Processing Units (GPUs) allow for parallel processing of data which means that the model training can be quickened for machine learning applications. For example, unlike with a CPU where the work is accomplished in a sequential manner, a GPU is capable of doing a number of tasks at the same time which makes the working process more efficient.
Managing Deep Learning Workloads
GPU complementing approaches are particularly suitable for large or deep neural networks which require convolutional layers and backpropagation computation. A cloud engineer deploying Generative Adversarial Networks or RNN (Recurrent Neural Networks) which is commonplace mostly depends on GPU server cluster systems for better performance.
HPC and Cloud Exploration
GPU servers coupled with cloud resources allow for multiple users or projects to access the same hardware enabling cooperation between projects. AWS EC2 P4 instances and Google Cloud also provided GPU instances are well-known tools that can be scaled.
What to Look for in a GPU Server Case
The selection of a correct chassis definitely affects how a server would operate and what its purposes would be. Consider the following design parameters in making a selection:
Basins and Slots for the Mounting of the White Drive.
Verify that the case has hot-swappable drive bays which will allow expanding subdivisions during upgrades. Sockets over PCIe Gen 4 are best accommodate the need for increased bandwidth, particularly on NVME modules or high bandwidth adapters when they are mounted.
Putting In Place 16 Lane PCI, When Going For The GPU
The support for up to x16 lanes of PCIe assures a much larger communication throughput between the installed GPU and other components of the PC which helps eliminate most communication bottlenecks especially during heavy calculations.
Customization Features For Different Server Requirements
Always look out for and consider purchasing server applications that include a modular design to facilitate capability for changes in GPU configurations, power supply units, memory limits, and cards for expansion. Optimal for data centers are servers that can use out-of-band management tools such as IPMI or Redfish.
How Does the GPU Server Impact Machine Learning and Development?
The Importance of High-Performance Compute Power
Researchers now have the needed high-performance computing power to experiment with larger models and solve problems in a novel fashion. To illustrate, GPT-4, alongside other natural language models, would now have GPUs capable of superior memory and computation management.
Overcoming Issues In The Training Of Large AI Models
Training models such as CNNs or Transformers requires adjusting thousands of parameters. With GPU servers, it is possible to portray multiple times with ease, which accelerates the development process on steroids.
What Are The Real World Use Cases of The Technology
A few prominent use cases of GPU servers include:
Medical Imaging:
– using MRI and diagnostic imaging.
Speech Recognition:
– Real-time transcription of language in the required context.
Autonomous Vehicles:
– Provides AI-based decision-making power along with LiDAR integration.
Your GPU Server Case Design and Multi-Usability
Your GPU Server case design needs and AI developer considering that you train models, an HPC manager, IT professional and a Cloud engineer enabling you to max out on computation complexity. A well designed GPU server boosts efficiency and eases procedures. Move up with carefully concurrent GPU systems to make the most of your productivity potential.